Generative Artificial Intelligence: Transforming Content Creation and Beyond
Generative Artificial Intelligence (AI) refers to algorithms capable of creating new content, such as text, images, audio, and code. Recent breakthroughs in this field have the potential to radically transform content creation and various industries. Tools like ChatGPT, GPT-3, and image generators such as DALL-E exemplify how generative AI is pushing the boundaries of what machines can achieve.
The Evolution of Generative AI
Since ChatGPT’s debut in November 2022, generative AI has advanced significantly. New tools, regulations, and technological improvements are continuously emerging. Despite initial fears, the potential benefits of AI are becoming increasingly evident. Machine learning, the backbone of AI, has already demonstrated its impact in areas like medical imaging and weather forecasting. A 2022 McKinsey survey highlighted that AI adoption has more than doubled over the past five years, with investment continuing to surge. Generative AI tools are set to revolutionize job functions across various sectors, though the full scope of their impact remains to be seen.
Understanding Generative AI
Generative AI encompasses models that produce new content by learning from vast datasets. These models are trained using large volumes of data to recognize patterns and generate outputs that mimic human creativity. Generative AI can create diverse content, including text, images, and even video. For instance, GPT-3 and its successor, ChatGPT, are capable of generating human-like text, while DALL-E can produce unique images based on textual descriptions.
Machine Learning vs. Artificial Intelligence
Artificial Intelligence (AI) involves machines performing tasks that typically require human intelligence. Machine learning, a subset of AI, involves models that learn from data without explicit human programming. Traditional machine learning techniques include statistical methods and predictive models, which observe and classify patterns. In contrast, generative AI goes a step further by creating new content rather than merely classifying existing data.
Generative AI Models: How They Work
Text-based generative AI models like ChatGPT use self-supervised learning, where they predict text sequences based on large-scale datasets. These models are trained on diverse internet content, allowing them to generate coherent and contextually relevant text. The quality of outputs depends on the model’s training and its ability to generalize from the data it has seen.
Building Generative AI Models
Creating a generative AI model is resource-intensive, often requiring significant computational power and data. Companies like OpenAI, DeepMind, and Meta have the resources to develop such models, investing millions of dollars in training them on extensive datasets. For example, GPT-3 was trained on approximately 45 terabytes of text data, a process costing several million dollars. This level of investment is typically beyond the reach of smaller startups.
Applications and Outputs of Generative AI
Generative AI can produce a wide range of outputs, from realistic text and images to code and simulations. For instance, ChatGPT can generate detailed essays or creative content, while DALL-E can create intricate images based on text prompts. The outputs can sometimes be surprisingly accurate and lifelike, though they may also include errors or biases. For example, DALL-E’s Thanksgiving image with lime-garnished turkey demonstrates both the creative potential and limitations of current models.
Potential Uses and Benefits
Generative AI presents numerous opportunities for businesses. It can rapidly generate written content, assist in code development, and create marketing materials. In industries like healthcare, generative AI can enhance image resolution or analyze complex datasets. By saving time and resources, generative AI enables organizations to explore new opportunities and create additional value.
Challenges and Limitations
Despite its promise, generative AI has limitations. Outputs may occasionally be incorrect or biased, reflecting the data on which models are trained. For example, biases inherent in internet data can influence AI-generated content. Additionally, generative AI models can be misused for unethical purposes or produce inappropriate content. Addressing these challenges requires careful management of training data, the use of specialized models, and ongoing human oversight.
The Future of Generative AI
The field of generative AI is rapidly evolving, with ongoing research and development likely to bring new innovations. Future advancements may include more sophisticated models, better handling of biases, and integration into various business and personal applications. As generative AI becomes more integrated into daily life, regulatory frameworks will also need to evolve to address new risks and opportunities.
Conclusion
Generative AI is poised to transform content creation and various industries. While the technology holds significant potential, it also presents challenges that must be managed carefully. By understanding the capabilities and limitations of generative AI, organizations can harness its power effectively while mitigating associated risks. As the field continues to develop, staying informed and adaptable will be crucial for leveraging generative AI’s full potential.
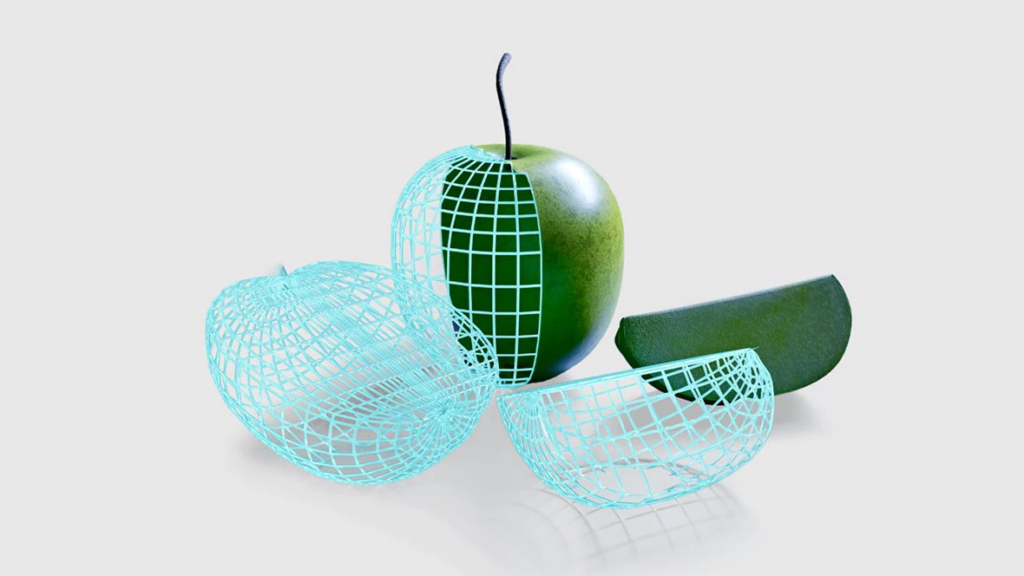